AI Delta Learning, optimising artificial intelligence for autonomous driving
When changing the sensor technology or the environment, the neural networks of autonomous vehicles have to be trained from scratch. The AI Delta Learning project aims to simplify this process, making autonomous driving even closer.
In an autonomous vehicle, artificial intelligence has to learn to recognise its surroundings and is therefore 'trained' by viewing a lot of images. The training process has to be modified and customised according to the peculiarities of each operational area.
Stop signs, for example, look similar in many countries, but in others - such as Japan, China and Algeria - they are different from those used in Europe or America. For human drivers, these differences are easily understood and assimilated, while artificial intelligence has to be trained from scratch again: a process that takes a long time, is expensive and slows down the global development of autonomous driving.
A joint project for artificial intelligence
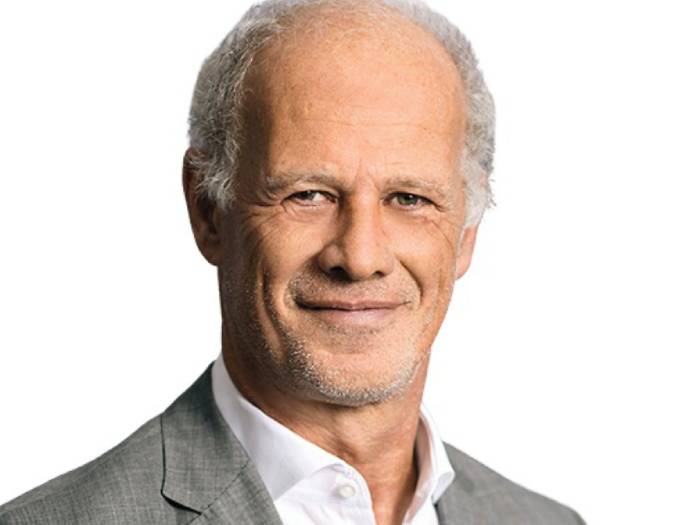
The AI Delta Learning project aims to develop a method to train autonomous vehicles selectively. Its importance is demonstrated by the participants and the funder, which is the German Federal Ministry of Economic Affairs and Energy. Partners include Porsche Engineering, CARIAD, BMW, Mercedes-Benz, major suppliers such as Bosch, and nine universities, including the Technical University of Munich and the University of Stuttgart.
The aim is to reduce the effort required to be able to infer things from one driving situation to another without having to train AI specifically for each thing.
The AI family for autonomous driving
This project is part of the AI family, a flagship initiative promoted by the German Association of the Automotive Industry aimed at advancing connected and autonomous driving. Since January 2020, around 100 people from 18 different partners have been working on AI Delta Learning, to build a catalogue of methods that can be used to enable knowledge transfer in artificial intelligence.
In autonomous vehicles, the neural networks that interpret traffic are similar to the human brain but differ in some crucial points. One of these is that they can only acquire their abilities all at once, typically in a single large training session.
Complex situations and domain changes
In the development of autonomous driving, even trivial changes require massive efforts. For example, replacing cameras with a resolution of two megapixels with better models (i.e. eight megapixels), means that the artificial intelligence has to be re-trained with millions of snapshots from traffic again in order to recognise objects at the higher resolution. The same thing happens if a camera, sensor or radar is positioned just slightly differently.
Experts call this a 'domain change': from driving on the right to driving on the left, from sunshine to snow, and so on. Human drivers adapt easily thanks to intuition and transfer their knowledge to the changed situation. Neural networks are not yet able to do this: if an unfamiliar object such as an e-scooter appears in traffic, the software must familiarise with it in order to be fully operational.
Teaching change
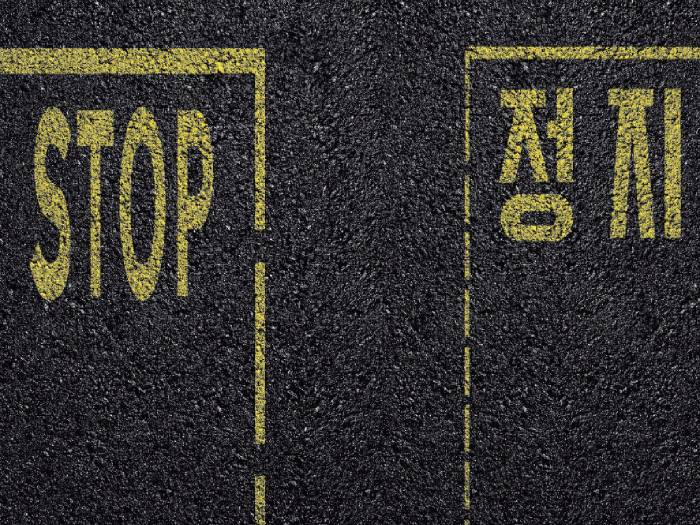
So far, it has not been possible to teach the algorithm only 'delta', i.e. change from what has already been acquired. In order to become familiar with a new scenario it is necessary to enter a complete data set, a bit like a student having to go through the whole dictionary every time they learn a new word.
Obviously, this is a learning process that uses enormous resources: it currently takes 70,000 graphics processor hours to train an autopilot. Even if many graphics processing units (GPUs) are used in parallel to 'train' the neural networks, the effort is still considerable.
Manual work on data
In addition, neural network needs annotated images, that is images from real traffic events in which important elements are marked, such as other vehicles, lane markings, or crash barriers. Manually completing all the notes in a snapshot from city traffic takes at least an hour; the process can also be partially automated, but large computing capacities are required.
Moreover, when it has to adapt to a new scenario, in some cases a neural network can forget what it has learned. A concrete example: an AI module trained with US traffic scenes (empty highways and vast horizons) could reliably identify the sky. When the same module was trained with a German dataset, it had problems identifying the sky in the American images, because in the German ones it was often cloudy or buildings blocked the view.
The importance of data
In cases like this, the module must necessarily be retrained from scratch with both datasets - a time-consuming process that is limited by storage space. But sometimes very representative images are enough to "refresh the knowledge": in this case, instead of showing the module all the American and German road scenes again, a few dozen images with typical motorway views were selected and this was enough to remind the algorithm what the sky looked like.
It is precisely such optimisation possibilities that AI Delta Learning aims to find. The project is divided into six application areas in which each partner seeks a method to speed up and facilitate training. Proven solutions are shared among the organisations involved.
Knowledge distillation
A further promising approach is for two artificial intelligence systems to train each other. First, a teacher model is built, it receives training data in which a class of objects, for example signs, is marked. The second AI system is the student model, which receives a dataset with other classes of objects marked, such as vehicles, roads, trees.
Then the process begins and the two AIs learn new concepts by exchanging roles. This method is known as 'knowledge distillation' and could make vehicle customisation much faster. When a car is to be introduced in a new market, it’s enough to use a different teacher model for the local signage and everything else remain the same.
Experimental phase
Most of the current work is experimental and it is not possible to predict which method will be best. Probably, the optimal solution will be an intelligent combination of different methods. Considering the progress made so far, it is plausible to expect to show the first methods for AI Delta Learning at the end of 2022, i.e. when the project ends, with significant benefits for the entire automotive industry.
Potentially, according to an expert’s opinion, the automation of the AI training chain through AI Delta Learning could lead to a halving of human labour input in the development of autonomous vehicles.
Source: Volkswagen AG
VGI | Responsible OU: VP | Creation date: article date | Class 9.1